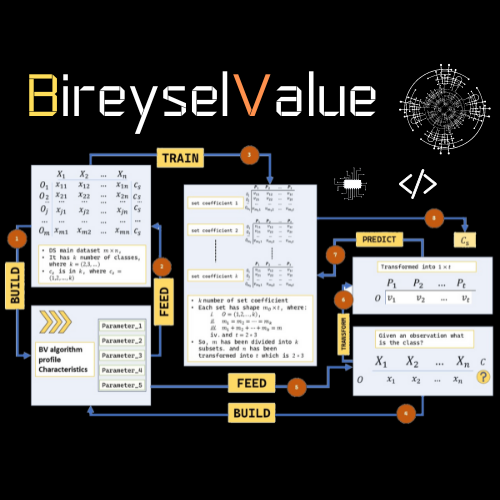
The BireyselValue, a Proposed Method for Solving a Classification Problem.
This paper presents a new method for solving a classification problem; the BireyselValue method assumes that the individual traits of a class help to classify an observation based on similarity measures. The method involves three stages to solve the classification problem: the building stage, the training stage, and the prediction stage. The first two stages accomplish two key steps: firstly, five parameters are used to transform any observation of size 𝑛n variables into six variables; secondly, subsets of the individual traits of each class are created. As a result, the parameters, the subsets of the individual traits, and a scaled version of the training dataset are saved as a predictive model. Ultimately, the prediction stage uses the elements in the predictive model to transform the observations that are to be classified and of size 𝑛n into the size of sixvariables and to perform similarity measures between the observation and the individual traits of class to make the final prediction. The experimental results obtained on 6 multiclass datasets from different domains showed that the proposed method is efficient at solving classification problems. Moreover, the method can potentially be used for purposes other than solving a classification problem.
“The Norm Culture” advocates for the introduction of a security layer in continuously learning AI models to protect against data and label poisoning attacks.
This paper presents a method to protect learning AI models against data and label poisoning attacks; The Norm Culture method posits that each class in an image classification problem possesses an inherent structure that serves as a primary defense against attacks—such as data or label poisoning—that can corrupt new training and testing samples during the parameter update phase of an AI predictive model within a conventional deep learning framework. The method requires calculating three elements from the essential training and testing samples. The first element is the flattened matrix representing the class image. The second element is the class alpha, a scalar that represents the weight norm of the class. The final element is the most recently validated AI predictive model. The experimental outcomes on a binary class image classification dataset from health domains indicate that the proposed method effectively identifies training and testing sample images compromised by either type of attack one or two. Additionally, there is potential for enhancing the method within the mathematical functions of the AI framework.
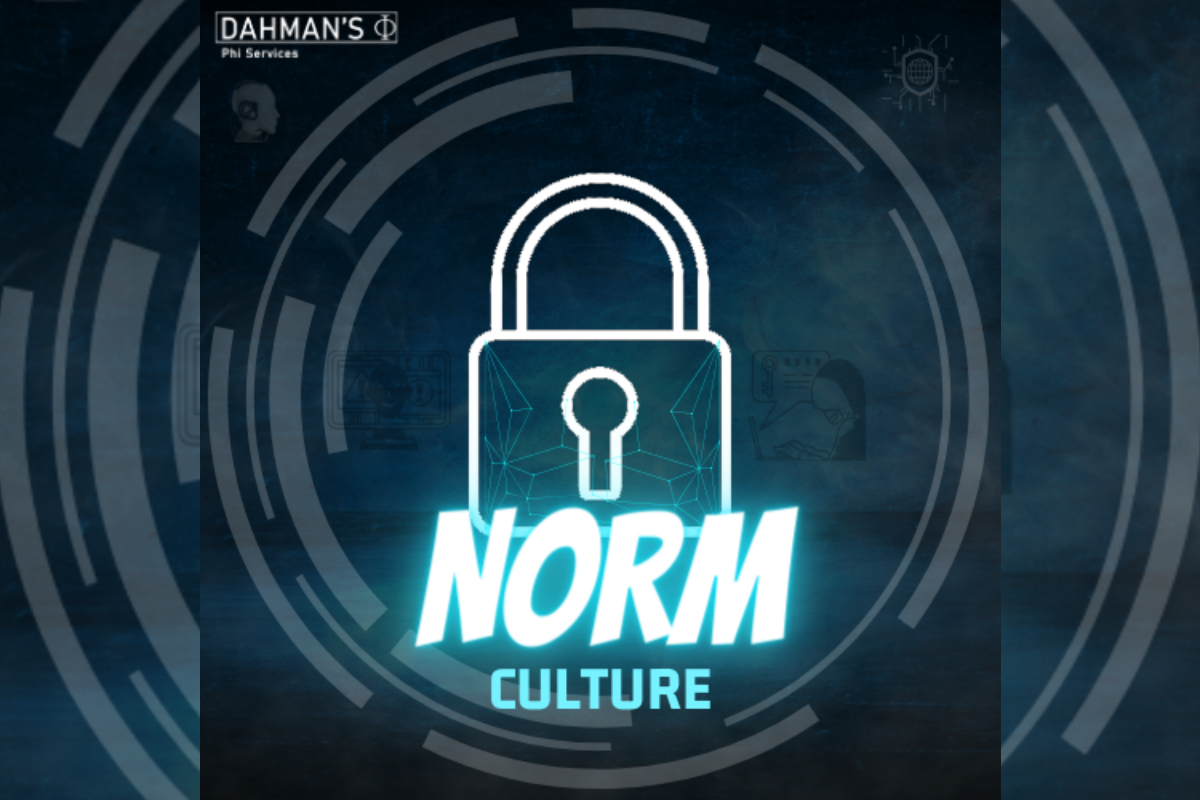
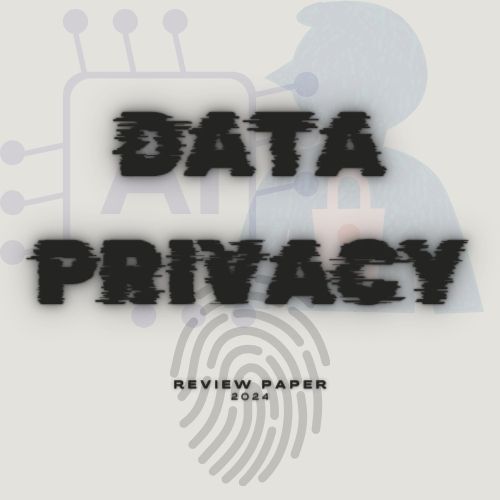
Review of Data Privacy Techniques: Concepts, Scenarios and Architectures, Simulations, Challenges, and Future Directions.
The recognition of data as a natural resource has made headlines in the new era of industrialization. Companies now leverage this resource to enhance their services and products, often promising optimal outcomes. While data has always held significant value, recent advancements in AI and ML frameworks have brought this fact to the forefront. However, it was not until major scandals involving large corporations came to light that the critical issue of privacy was remembered. Consequently, the intersection of data, AI and ML frameworks, and privacy has emerged as a new area of research. Although numerous works have reviewed the development of various data protection techniques, it seems that most of them address the subject from a single perspective or attribute the entire concept of data privacy to a specific technique. This review aims to present an overarching view of the topic. It offers a systematic guideline that establishes a proper connection among the three elements: data, AI & ML frameworks, and privacy. The paper delves into each element from both an abstract and concrete standpoint, presenting the latest techniques to address data privacy concerns, including numerous lab simulations. It also recommends tools and resources for further study. Ultimately, it wraps up the central topic by outlining the challenges and prospective future research directions.
”DisguiseData” A Proposed Method for Creating
Synthetic Data Based on The Geometric Structure of
The Original
Data holds a crucial role in the standard system of input, processing, and output, particularly representing the input. With high-quality data, there is an assurance of a smooth process and an effective outcome. However, the issue of privacy in data acquisition has become a significant concern in recent years, forming the core of numerous research studies. This paper introduces a novel approach to generating synthetic data that retains the same statistical structure as the original, based on the geometrical representation of each data point. This newly generated synthetic data can act as a substitute for the original, ensuring the integration of privacy into any application utilizing the data. Experimental results on two datasets of varying sizes demonstrate that the proposed method can effectively produce synthetic data with the same statistical structure and a comparable level of accuracy for predictive tasks as the original data.
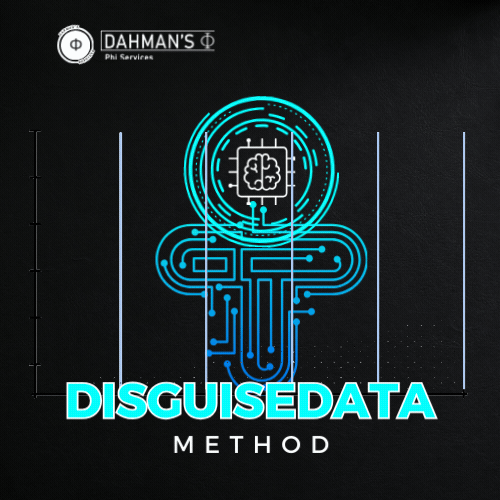